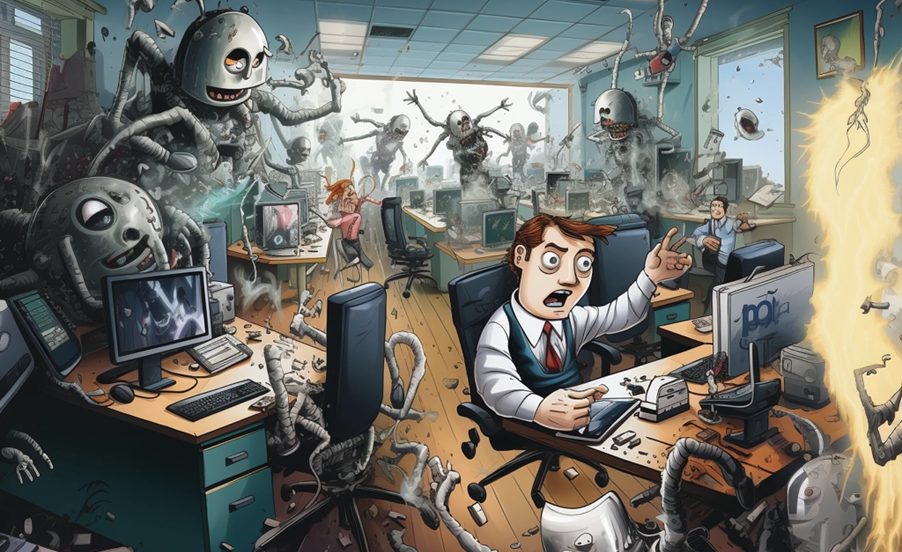
GenAI risks: Mild, Medium or Extra Hot?
How should we think about “risk” when it comes to GenAI, and what should you do about it? In part one of a two-piece series, we look at one way of thinking about classifying the risk from GenAI workflows. Part two covers how to mitigate those risks while safely and responsibly deploying GenAI tools.
One of the most incredible aspects of GenAI is the seeming ability to start using it with just the briefest of introductions. Tools like OpenAI’s ‘ChatGPT’ chatbot and ‘DALL-E’ image generator allow a user to load a browser window, type in a short instruction and immediately receive something useful back. It would be entirely possible to bring GenAI into your daily workflow with the minimum of effort, right now, and potentially many of your colleagues already have. But with all the excitement, and the genuine potential for transformative impact, as with any new technology there’s always going to be a nagging thought “just how risky is this?”. Responding to this, some organisations took the early approach of banning GenAI usage outright, while in other cases strict limits have been placed on the kind of data that can be used in GenAI tools. But what’s the best approach to take?
Before we start, let’s put the current risks from GenAI in perspective. While the AI chatbot used to create the above image wanted to add the caption of “GenAI: The power to create…or destroy!”, we aren’t yet at the point where humanity is under direct threat of annihilation. The sort of risks we are considering here are more prosaic, albeit hugely important:
- Security: You don’t want to have your employees accidentally upload sensitive corporate documents to a public GenAI service and then find they can’t get them back
- Privacy: You don’t want to inadvertently open up sensitive employee data and make it easily accessible to anyone in your organisation
- Ethics: You don’t want to introduce an automated process that later turns out to be error-prone, depriving people of benefits/entitlements to which they are due
Is it worth it?
Given the risks articulated above, you may be tempted to ignore GenAI altogether, deciding to wait it out until the dust settles. This probably isn’t the right approach for a few different reasons. Firstly, you’ll be waiting a long time. GenAI is not like other technologies in that the speed of development and the evolution of tooling is occurring so rapidly that new versions are released every couple of months. This means that it’s not likely to be the case that you can do a one-off risk assessment and move on, with GenAI there needs to be continuous evaluation. Given this is the case, there’s minimal benefit to waiting. Secondly, GenAI isn’t going anywhere, and the benefits being touted are likely to be transformational. By waiting you are effectively ceding ground to competitors, and in all likelihood throwing away resources that could be utilised more efficiently via GenAI tools. Lastly, nobody is proposing a blasé attitude to utilising GenAI, there are better and worse ways to deploy these tools, and caution is advised. Look out for our upcoming articles on understanding GenAI organisational maturity.
Not all GenAI cases are created equal
So let’s assume you’ve decided to move forward with some GenAI use cases, and you’d like to know what risks are present and how to mitigate them. Perhaps you are in the fortunate position where you already have organisational policies and guardrails that set out what you can and can’t do. In all likelihood, you don’t have this. Even if you do, many policies and guidelines give the impression that AI-powered use cases should all be lumped together (for instance, banning any use of personal data). This seems unhelpful, as it ignores the context of the use case and how information is to be used. There’s a big difference between using a GenAI tool to process personal council tax data for the purposes of deciding eligibility for benefits/exemptions and using it to craft more easily understood letters for citizens.
The three-tiered system for AI use cases
An alternative idea would be to look at the approach proposed by the European Union (EU) and some other organisations, based upon traditional risk management approaches of graded risks. The EU is suggesting a three-tiered system where AI use cases are categorised with corresponding actions that need to be taken against each tier:
Extreme Risk: A use case that poses unacceptable risks to citizens, and should therefore be prohibited
High Risk: A use case that has the potential to cause harm, and is therefore subject to a series of mandatory safeguards
Limited Risk: A use case that does not influence the outcome of decision-making, and is therefore not subject to safeguards (though they may still be recommended)
Here are some examples:
You can see that this approach is primarily focused on the risk to people, but it would be equally possible to extrapolate the categories to include organisational risk factors.
So what are the factors that determine which ‘tier’ of the risk grading system applies? The approach taken by the EU is primarily outcome-focused. That is, largely ignoring how the technology works and the data that is needed, and instead focusing on the intended outcome from the system. This means that the risk system ends up feeling like a catalogue, with lists of potential use cases that can be refined over time. Here are some examples (not comprehensive, the EU lists many more):
Extreme: Generally anything that is illegal, or runs contrary to expected norms and human rights. E.g. Using AI to introduce manipulative/subliminal techniques with the aim of getting citizens to act in certain ways. Using AI for biometric classification (e.g. scanning facial features to impute sexual orientation). Using AI for emotional state prediction of workers.
High: Generally anything where decisions will be made that impact people. E.g. Public authorities using AI to evaluate the eligibility of citizens for benefits and services. AI systems intended to be used for recruitment or selection (advertising vacancies, screening applications, evaluating candidates via interviews or tests)
Low: Use cases where a human has already done the bulk of the work, or where there is no real impact on decision making. E.g. Transforming unstructured data into structured data, improving the language used in previously drafted documents, translation of documents. An interesting example that’s counted as limited risk: “Given a certain grading pattern of a teacher, [AI] can be used to check ex post whether the teacher may have deviated from the grading pattern so as to flag potential inconsistencies or anomalies.”
When thinking about assessing your own risks, explicitly listing out the kind of use cases you may wish to pursue has certain benefits (primarily that it’s explicit to users of GenAI what they can and can’t do), but it also means that any new use case needs to be ‘slotted in’ to the risk framework, which potentially introduces a governance burden for every use case.
This is the obvious downside with the “catalogue” approach, in that it can’t possibly cover everything. An alternative would be to keep the three tiers but to unpick the broader features of these use cases and come up with generalisable guidelines, i.e., what exactly is it that makes an “extreme” use case unacceptable (e.g. it’s illegal). The downside of this approach is that it does risk users misinterpreting the guidelines.
Next Steps
Either way, let’s say you’ve got your risk grades, and your list of potential use cases. Now what? What does it mean for something to be “high risk” vs “limited risk”, what do you do about it? For that, you’ll need to read part two.
If you want expert help in understanding how GenAI could, and should, be rolled out across your business Global IT Solutions is on hand to help. Contact us at [email protected].
Join our GenAI discussion group to access more content like this one and be part of our community of leaders.